Smart investments: AI disruption in the financial market
- Marcelo Firpo
- May 8
- 7 min read
Updated: May 9
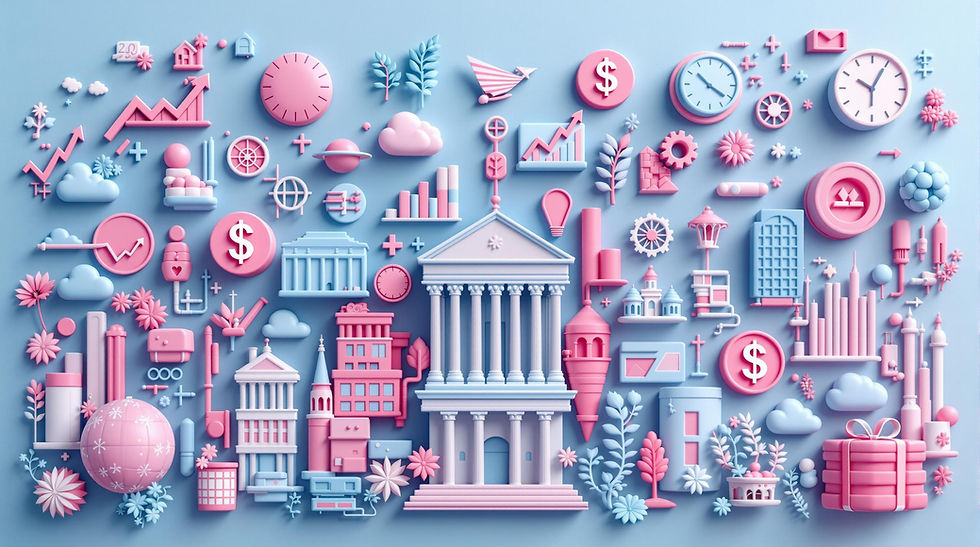
AI-generated summary:
In the financial market, technologies such as Machine Learning and Generative AI are being used in areas such as fraud prevention, risk management, service personalization, and market analysis. Financial institutions that adopt these solutions see gains in efficiency, cost reduction, and increased revenue. In addition, AI facilitates regulatory compliance, optimizes customer service, and improves portfolio management.
The advancement of Artificial Intelligence (AI) is redefining the financial market, bringing innovations ranging from process automation to advanced data analysis for more accurate forecasts. Technologies such as Generative AI (GenAI) and Machine Learning (ML) are transforming the way banks, brokerages and investors make decisions, driving efficiency and reducing risk. In this dynamic scenario, understanding how these tools are shaping the sector becomes essential for companies and professionals who want to remain competitive.
AI in the financial market: the basis of a new standard of excellence
Adopting AI in the financial sector is no longer optional, but a strategic necessity. Financial institutions are increasingly investing in AI to automate tasks, analyze large volumes of data, and offer more personalized services to customers. NVIDIA’s “State of AI in Financial Services” report, published in February 2025, indicated that more than 60% of companies in the sector reported an annual cost reduction of at least 5% thanks to the implementation of AI-based solutions. In addition, the same study highlighted that almost 70% of respondents saw a revenue increase of 5% to 20% due to the adoption of AI.
"At BlueMetrics, we believe that artificial intelligence, as long as it is based on a well-balanced data structure, is the key to leveraging the financial sector. Our mission is to enable companies to use the power of AI to optimize processes, reduce costs and offer exceptional customer experiences," says Denis Pesa, CEO of BlueMetrics.

Some applications of AI in the financial sector
1. Corporate Records and Market Intelligence
AI makes it easier to collect and analyze corporate data, ensuring financial institutions maintain accurate and up-to-date records. Additionally, Machine Learning (ML) techniques identify market trends and provide valuable insights for strategic decision-making.
Tiger Brokers, a Chinese brokerage firm, has integrated the DeepSeek-R1 AI model into its chatbot, TigerGPT, enhancing market analysis and trading capabilities. This innovation has provided clients with deeper insights into their investment decisions.
2. Production of ESG Strategies and Initiatives
AI can be used to develop and monitor strategies related to environmental, social and governance (ESG) factors. Advanced algorithms analyze sustainability data and help create initiatives that meet ESG criteria.
Capgemini uses generative AI and cloud solutions to drive sustainable transformation in businesses. These technologies help improve competitiveness, productivity and sustainability, while aligning corporate operations with ESG criteria.
3. Claims Assessment, Fraud and Irregularity Detection
Fraud detection and prevention are critical areas where AI has excelled. ML algorithms monitor transactions in real-time, identifying suspicious patterns and preventing fraudulent activity.
Banco Original implemented an AI platform in partnership with IBM, using chatbots for customer service and facial biometrics to validate high-value transactions. These initiatives have resulted in greater security and efficiency in banking operations.
4. Agility in the Subscription Process, Review and Comparison of Policies
Automation of underwriting and policy review processes is another area where AI can benefit. ML models can quickly analyze large volumes of data, streamlining credit approval and risk assessment.
Upstart, a US fintech, uses ML to improve its credit underwriting processes. The company has developed a prototype that predicts invoice payments with up to 77% accuracy, improving customer prioritization and supporting collectors’ daily work.
5. Customer Service Support
AI-powered chatbots and virtual assistants provide immediate support to customers by answering frequently asked questions and resolving common issues. This improves the user experience and frees up human resources for more complex tasks.
Banco Original implemented the "Original Bot", a digital tool that interacts with customers via Facebook Messenger, providing programmed responses on various banking services. This initiative resulted in more than 1 million monthly chatbot services, increasing efficiency and customer satisfaction.
6. Documentation and Management of Risk Models
AI helps create and maintain more accurate risk models by analyzing historical data and identifying patterns that may indicate potential financial problems. This enables more proactive and informed risk management.
Raiffeisen Bank International (RBI) has collaborated with Reply to develop a portfolio optimization solution using the concept of Quantum Annealing. This innovative approach has enabled the efficient analysis of large volumes of data, improving accuracy in risk management and investment decision-making.
7. Automated Data Ingestion for Quantitative Analysis
AI enables the automation of financial data collection and structuring, speeding up quantitative analysis and eliminating time-consuming manual processes. This enables faster, more informed decisions for traders, analysts, and investment managers.
JPMorgan Chase has developed the COiN (Contract Intelligence) platform, which uses machine learning to analyze and extract critical data from legal documents. This innovation has significantly reduced the time required to process and interpret large volumes of data, increasing efficiency and accuracy in financial operations.
8. Portfolio Management and Next Best Action
The use of AI in portfolio management enables the personalization of investment strategies based on the risk profile and preferences of clients. Additionally, ML algorithms help predict the next best action for each investor.
Spanish company Renta 4 has implemented AI systems to optimize investment portfolio management by analyzing large volumes of market data and adjusting strategies based on real-time economic conditions.
9. Policy and Regulation Search Engine
AI is making it easier to access financial industry regulations and standards, helping companies stay compliant with legal requirements. AI-powered systems can process and interpret large volumes of regulatory text in seconds.
HSBC has implemented AI tools to monitor and interpret global regulatory policies, ensuring compliance with different legislation and avoiding sanctions risks. These automated solutions enable the bank to quickly adapt to regulatory changes while maintaining compliance efficiently.
10. New Customer Onboarding Process
Automating customer onboarding reduces friction in the account opening process and improves the user experience. AI and biometric recognition ensure fast and secure identity verifications.
German digital bank N26 uses digital onboarding processes that allow customers to open an account in minutes, directly from their smartphone. This innovative approach speeds up identity verification and improves the user experience, reflecting the efficiency that AI brings to the financial industry.
Want to see GenAI, Machine Learning, and data solutions making a difference in your company?
The role of data and language models
The Importance of Data Pipelines in the Finance Ecosystem
A well-structured data pipeline is essential to ensure that AI models operate efficiently and securely. In the financial sector, where data accuracy and security are crucial, effective data pipeline management directly impacts the quality of predictions and analyses generated by AI.
Main steps of a data pipeline:
Data Ingestion
Collecting information from internal databases, financial market APIs, and external sources such as economic news.
Processing and Transformation
Cleaning and structuring data to ensure the quality of the analysis.
Secure Storage
Use of data lakes and cloud solutions to manage large volumes of information.
Distribution and Consumption
Integration of processed data into risk management, economic forecasting and credit decision systems.
"With AI and well-structured data, it is possible to transform financial management and strategically reduce risks", adds Gabriel Casara, CGO of BlueMetrics.
Language Models (LLMs): increasingly decisive
Large Language Models (LLMs) are fundamental in improving the customer experience and in the way financial institutions manage information and interactions with their different audiences and partners. Models such as GPT and BERT enable the automation of complex tasks, from the analysis of financial documents to the generation of market reports.
Text Generation
Used in chatbots and virtual assistants, LLMs provide quick and accurate answers to financial queries.
Sentiment Analysis
They monitor opinions about the company and market trends from social networks and financial news.
Report Automation
They reduce the time spent on reporting, generating valuable insights from large volumes of data.
Additionally, AI models combined with Retrieval-Augmented Generation (RAG) enable up-to-date information to be incorporated in real time. This means that enterprise virtual assistants can provide accurate answers based on the latest financial data, and legal research systems can streamline regulatory and regulatory queries in the financial sector.
The ability of language models to process large amounts of data quickly and accurately is essential for banks and financial institutions that deal with a massive flow of information daily. From personalizing customer service to detecting fraud, these technologies are making financial operations safer and more efficient.
How BlueMetrics makes a difference for the financial sector
BlueMetrics has stood out in the financial market by providing innovative solutions based on Generative AI and Machine Learning. With more than 160 projects delivered to over 70 clients in Brazil and the US, the company has helped financial institutions implement AI strategically, accelerating processes and optimizing operations.
BlueMetrics' main deliveries for the financial sector include:
Automations : implementation of virtual assistants and chatbots to improve customer experience and reduce operational costs.
Fraud prevention models : advanced solutions that identify suspicious transactions in real time, reducing financial risks.
Risk analysis optimization : development of ML models to assist in credit decision-making and risk management.
Regulatory process improvement : AI-based tools to ensure compliance with financial and regulatory standards.
Regarding the importance of AI for the financial sector, Denis Pesa highlights: "Generative Artificial Intelligence and Machine Learning are determining factors for competitiveness in the financial market. The ability to analyze data on a large scale, personalize services, and prevent fraud in real time puts institutions that adopt these technologies ahead of the competition."
With AI and efficient data management, financial institutions can operate with greater precision, security, and innovation, ensuring an increasingly digital and agile future.
Conclusion
The transformation brought about by Artificial Intelligence in the financial sector is an irreversible path, where an innovative mindset and advances in technology go hand in hand to shape a more efficient, secure, and personalized market. As financial institutions continue to adopt these solutions, new opportunities arise for the creation of services and products that add value to both companies and customers.
However, the real difference will lie in the ability to combine the strategic use of AI with robust data governance and an organizational culture that values adaptation and ethics. Success in implementing AI is not limited to efficiency gains or cost reductions, but rather to an exponentially better experience for customers, as well as gains in terms of security and process governance.
If you work in a financial services company and want to explore the immense potential of AI, we are ready to talk and build your next project together! 🚀
Let's talk about it?
Discover some Use Cases.
Comments